Sampling
Introduction
Sampling plays an important role in the field of statistics and we should know the basic concept of it. Sampling is a method used in statistical analysis of a data where a specific number of samples are taken from a population for a study. In the case of an infinite population, it is not possible to do on the whole population. In such cases, a sample study is the only applicable method. For e.g. a manufacturer industry wants to check the quality of a product. It is not possible to check on each and every item is manufactured. So we will take some part of it for inspecting the quality.
There are different techniques of sampling used for different purposes. Some of the sampling methods are random sampling, stratified sampling and cluster sampling. But sometime due to time constraints or it could be similarities in data – we could not analysis the whole data. So in such circumstance we can apply sampling.
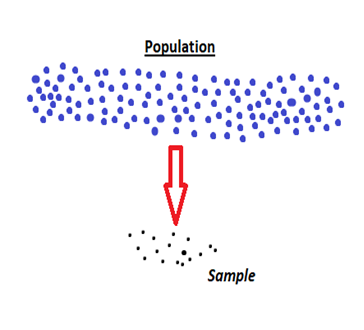
Sampling Methods & its applications
- Simple Random Sampling – It is a process of drawing a sample in such a way where every unit has an equal chance of being included in the sample. In simpler words, a process of selecting a sample randomly from a population.
For example, an engineer wants to take a sample of machine parts manufactured during a shift at a given plant. Since the parts from which the engineer wants to make the sample are manufactured during the shift at the same plant, it is quite safe to assume that all parts are representative.
Hence, in this case, a simple random sampling design should be appropriate.
- Simple Random Sampling – It is a process of drawing a sample in such a way where every unit has an equal chance of being included in the sample. In simpler words, a process of selecting a sample randomly from a population.
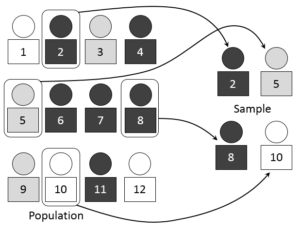
- Stratified Random Sampling – Here, the population is divided into a number of subgroups (called as strata) and from each stratum (Homogeneous in nature) a simple random sample may be selected to form the required sample.
For example, if the sample is taken from a population of parts manufactured either in different plants or in different shifts, then this technique can be more appropriate than SRS. Suppose, if the machine parts are manufactured in plants located in different parts of the country, then this technique can be beneficial.
- Stratified Random Sampling – Here, the population is divided into a number of subgroups (called as strata) and from each stratum (Homogeneous in nature) a simple random sample may be selected to form the required sample.
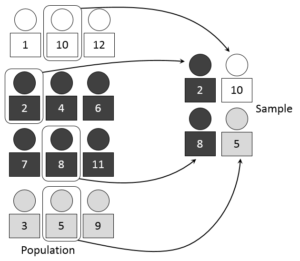
- Systematic Sampling – Systematic sampling is a procedure of drawing a sample in systematic way. It is obtained by selecting every Kth (K is known as sampling interval) individual from the population. This is particularly used in manufacturing processes, when the sampling is done from a continuously operating assembly line.
Suppose, a company wants to do sampling on a bulbs manufactured on a day to check whether it is achieving its quality standard or not (from a continuous process line). The systematic sample is spread more over the entire population than stratified sample because in stratified sampling the samples in the strata are drawn separately. This adds precision in some cases.
- Systematic Sampling – Systematic sampling is a procedure of drawing a sample in systematic way. It is obtained by selecting every Kth (K is known as sampling interval) individual from the population. This is particularly used in manufacturing processes, when the sampling is done from a continuously operating assembly line.
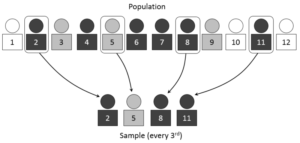
- Cluster Sampling – Cluster Sampling is a procedure of drawing sample from a randomly collected group of individuals. Here, the population is already broken into groups (clusters) and each cluster represents the population.
Suppose a company makes power banks, and like to test the effectiveness of the packaging. They don’t have a complete list, so simple random sampling doesn’t apply, and the power banks are already in boxes, so you can’t order them to use systematic sampling. And all the power banks are essentially the same, so there aren’t any characteristics with which to stratify them.
To use cluster sampling, a quality control inspector might select a certain number of entire boxes of power banks and test each power bank within those boxes. In this case, the boxes are the clusters.
- Cluster Sampling – Cluster Sampling is a procedure of drawing sample from a randomly collected group of individuals. Here, the population is already broken into groups (clusters) and each cluster represents the population.
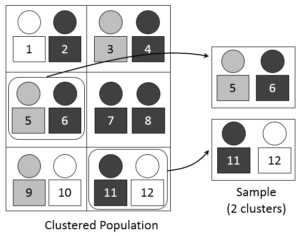
Benefits
- Representation of a sample.
- Accuracy of a data.
- Economical in nature.
- Less time consuming.
- Reliable outcomes.
Images – Referred from commons.wikimedia.org
Attend our Training Program, to know more about Statistics and Statistical Software. We conduct various training programs – Statistical Training and Minitab Software Training. Some of the Statistical training certified courses are Predictive Analytics Masterclass, Essential Statistics For Business Analytics, SPC Masterclass, DOE Masterclass, etc. (Basic to Advanced Level). Some of the Minitab software training certified courses are Minitab Essentials, Statistical Tools for Pharmaceuticals, Statistical Quality Analysis & Factorial Designs, etc. (Basic to Advanced Level).
We also provide a wide range of Business Analytics Solutions and Business Consulting Services for Organisations to make data-driven decisions and thus enhance their decision support systems.