Predictive Analytics
Introduction
We are living in a competitive world where everyone has to perform better than others. In business scenarios, an organization has to increase sales trend, enhances productivity, gain revenues and predict the future trends of particular business models. It helps businesses to remain competitive. Since we are living in a data-driven environment predictive analytics helps to understand the customers’ perspective and predict future sales. We can find its applications in many sectors like business, sales, airlines, healthcare, banking, etc.
Suppose we want to estimate growth in sales of a company based on current status. We have the past company data which indicates that the growth in sales is around one and a half times the growth in the economy. Using this insight, we can predict future sales of the company by using predictive analytics. Its highly recommended that Organisation’s in this Digital Age has a dedicated Enterprise Decisions Management system.
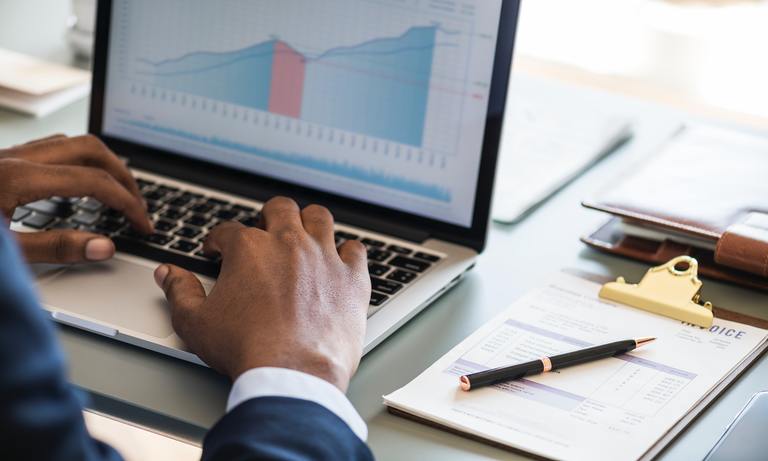
Popular Tools used in
Predictive Analytics
What is Predictive Analytics?
Predictive Analytics is a method of applying Statistical techniques combined with applied mathematics and computational science to predict and improve decision making strategy in given scenarios. With the application of predictive analytics – we could improve in Customer Acquisition, HR planning, sales strategies, policy making, financial activities, product pricing and so forth. Having tasted success with Predictive Analytics in some departments, organisations are now investing in a dedicated Enterprise Decisions Management system.
Common techniques used in Predictive Analytics
- Data mining – The process of extracting useful information from large sets of raw data is known as Data mining. It uses descriptive and inferential statistical analysis for analyzing the extracted data. Descriptive analysis is a method to analyze data that helps to describe, show or summary data. It consists of measures of central tendency and measures of dispersion. Inferential analysis is a method to analyze data for drawing inferences or make decisions about the population by using the sample data. Data mining uses methods like classification, clustering, association, etc. It would be helpful in business decision making processes.
- Machine learning – It is a “Field of study that gives computers the ability to learn without being explicitly programmed.” It identifies patterns from data and helps to make a decision with minimal human intervention. It comprises of supervised learning and unsupervised learning. In supervised learning, data are placed on the correct label and mainly prediction based on user input. We know the sample data relationships and based on it, we predict the outcomes. In unsupervised learning, data is neither labelled nor sorted and mainly focuses on discovering the patterns of data. The output is dependent on coded algorithms.
- Statistical Modelling – It is a mathematical model used for the prediction of a value. Generally, it is used on current data to predict what will happen next or to suggest actions to take for optimal outcomes. Regression analysis is the most common statistical modelling approach used in data analytics and it is the basis for more advanced statistical and machine learning modelling. It is a powerful technique in the field of statistical modelling used in prediction of the value of an unknown variable from a known variable, in predicting the value of one variable, given the value of another variable, when those variables are correlated to each other. Basically, regression analysis is used to predict an outcome based on historical data.
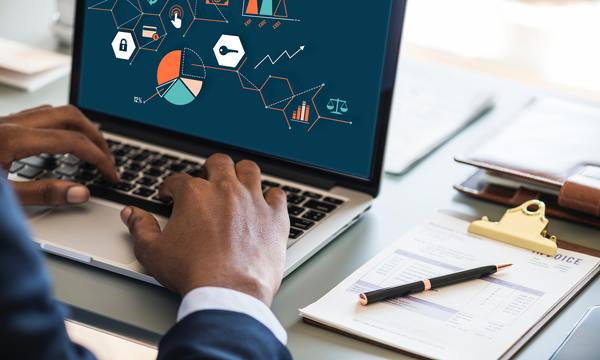
Some popular statistical methods used in Predictive Analytics
- Sampling – It is a process of taking a small set of observations (sample) from a large population. For e.g. population – a bag of rice & sample – a handful of rice. It is a common tool used in any form of data analytics. Some of the sampling methods are random sampling, stratified sampling and cluster sampling. But sometime due to time constraints or it could be similarities in data – we could not analyze the whole data. So in such circumstance, we can apply sampling.
- Correlation Analysis – It is used to study the closeness of the relationship between two or more variables i.e. the degree to which the variables are associated with each other. Suppose in a manufacturing firm, they want the relation between –
- Demand & supply of commodities.
- Production volume & the efficiency of machinery equipment.
- Statistical significance – It is a method to analyze data for drawing inferences or make decisions about the population by using the sample data (by sampling). For example, a manufacturer wants to check the product’s quality meets the pre-specified criteria. Here, it is not possible to check the whole population to make a decision. We collect sample data from the population. Based on the sample data, we need to make an inference for a population – This process is also called as “Hypothesis Testing”.
- Regression analysis – Regression analysis is a powerful technique in the field of statistical analysis used in prediction of the value of an unknown variable from a known variable, in predicting the value of one variable, given the value of another variable, when those variables are correlated to each other. Basically, regression analysis is used to predict an outcome based on historical data. The different types of regression analysis are linear regression, multiple regression, polynomial regression and logistic regression.
- Graphical Analysis – Here, the data are presented in the form of graphs or diagrams (mainly used for visualization). When we presented data through diagrams and graphs – it looks more convincing & appealing. Thus provide the meaningful outlook of a data. Some of the popular graphical tools used are
- Histogram
- Bar chart
- Pareto chart
- Scatter plot…
What role does Statistics play in Predictive Analytics?
Statistics is the foundation of predictive analytics. Since we now that predictive analytics is a combination of computer science and statistical models. There are various statistical methods which are the foundation of predictive analytics methods like sampling, hypothesis testing, correlation, regression and so forth. For the applications of these techniques, one should have a basic knowledge about it – “What are these methods mean?” and “Where and when we can apply?” Hence statistics plays a pivotal role in it.
Attend our Training Program, to know more about Statistics and Data Analytics Software (Minitab). We conduct various training programs – Statistical Training and Minitab Software Training. Some of the Statistical training certified courses are Predictive Analytics Masterclass, Essential Statistics For Business Analytics, SPC Masterclass, DOE Masterclass, etc. (Basic to Advanced Level). Some of the Minitab software training certified courses are Minitab Essentials, Statistical Tools for Pharmaceuticals, Statistical Quality Analysis & Factorial Designs, etc. (Basic to Advanced Level).
Also we provide comprehensive solution for Enterprise Decisions Management, for organisation interested in having a robust Decision Making process.